Observability has become a cornerstone of modern software operations—but let’s face it, it’s broken. Costs are spiraling, signal-to-noise ratios are off, and teams are stuck in a frustrating loop of over-instrumenting and then ripping it all out to save money. What if instead of just observing your systems, you could actually control them?
At ControlTheory, we believe it’s time to move beyond Observability to something more actionable: Controllability. Borrowed from the field of Control Theory, Controllability is about using feedback loops not just to watch your systems, but to actively shape and optimize their behavior. In this post, we’ll break down what Controllability really means, how it differs from traditional Observability, and the three pillars that make it work: Cost Control, Operational Control, and Adaptive Control.
What is Controllability?
Well, let’s start with was it isn’t. It isn’t continuing to send an ever increasing amount of telemetry data via 1 way “dumb pipes” to our existing observability vendors, who are happy to take it, and charge you for every byte ingested, stored or indexed. It isn’t a continuation of increasing MTTI and MTTR, which continue to go up, despite the aforementioned record levels of investment in our observability vendors. And it isn’t putting our development and engineering teams through a continuous cycle of knee jerk reactions, to remove instrumentation from our code to avoid cost overruns, only to add it back later because we need better insight – code changes which can have significant lead times, and absorb valuable cycles that could be used to move the business forward.
In short, current observability is broken, and we need to regain control of our observability data.
So what is Controllability really? How does it compare to Observability?
Well first up, it’s a longer word than observability (although both are long!) 🙂 Observability can be a mouthful to say, so it is sometimes abbreviated as “O11y” so Controllability in turn would be “C13y”!
On a more serious note, Controllability is an existing term that comes straight out of Control Theory – a field of engineering and mathematics that “deals with the control of dynamical systems in engineered processes and machines”, and “plays a crucial role in many control problems, such as stabilization of unstable systems by feedback, or optimal control.”. **** In fact, observability and controllability are “dual aspects of the same problem” to quote the 1st Wikipedia article linked earlier. We explored the differences between observability and controllability in a previous blog – in short control systems are relevant wherever we have feedback loops, and while observability deals with observing the state of the system, controllability is critical for altering the state of the system based on those observations, to drive to some desired outcome.
Here at ControlTheory, we view there as being 3 core outcomes we are trying to achieve – Cost Control, Operational Control and Adaptive Control.
Let’s dig into each of these a bit.
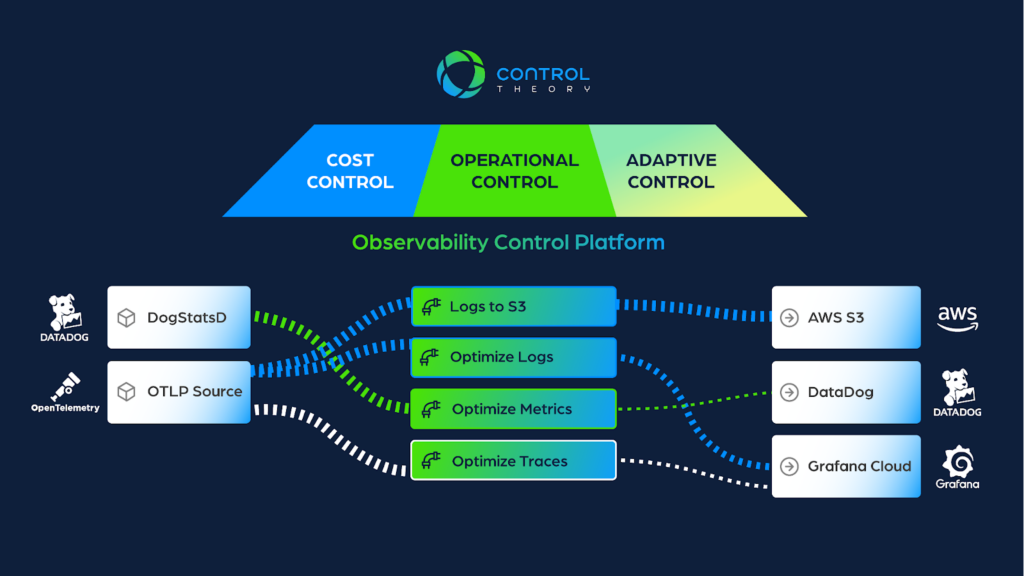
Cost Control
Top of mind for just about everyone with an Observability vendor in place today is regaining control over their soaring observability costs. It’s not uncommon when we talk to customers to find that they uncover sudden or unexpected changes in their telemetry only when they look at their bill, at the end of the month, or even at the end of the quarter! Awkward conversations with the CFO ensue, often leading to some of the “knee jerk” reactions we talked about above. There is a better way – using what we call “MetaMetrics” or the data about your observability data, we can get a proactive handle on underlying telemetry spikes or changes, and get attribution about where they’re coming from – what service, application or team is driving them – and were they caused by a recent code change?
We can then leverage additional active controls to mitigate these costs such as aggregation and filtering to control high metric cardinality or log volumes, or by routing telemetry to low cost “cold storage” like AWS S3, where it can be rehydrated later if we need it.
Open standards also play a crucial role in controlling costs, and while OpenTelemetry is making headway in democratizing our telemetry data and avoiding lock in on data collection, we also need to ensure our control planes and systems we use to control our telemetry data are also “built on open”, lest we jump from one lock in situation to another.
Operational Control
While cost gets a lot of the headlines, it’s really a symptom of a larger issue of asking “why?” we’re observing in the 1st place. For many teams, the “why?” is around shortening the process of root cause analysis (RCA), reducing our MTTI and MTTR, and generating meaningful business KPIs, but doing so while ensuring security, privacy and compliance of our observability data.
Controls like tail sampling allow us to separate the key signals from the noise (and cost), honing our engineering investigations into just those traces with high latency or errors, lowering MTTI and MTTR. Routing telemetry such as traces to cold storage like AWS S3 opens up new analytics possibilities, enabling us to analyze service to service latency or the customer experience over time (and releases), so we can answer the question “is our customer experience actually improving?” And this can all be done securely, through masking and redaction controls that ensure the right teams see the right telemetry data.
“MetaMetrics” are powerful here too, uncovering issues that many times go unnoticed, or as one customer put it “Individual ‘feature’ monitors didn’t trip, but the overall volume of data coming from the service raised eyebrows and allowed a faster response/remediation time.”
Adaptive Control
Finally, all of the controls above must be applied continuously, based on need, and driven through feedback loops. Due to the knee jerk reactions mentioned previously, development teams can become disincentivized to instrument key business applications and logic, for fear of cost overruns and reprisals. This is the opposite of what we want – we want our development teams to liberally instrument our applications for insights and better outcomes. Using adaptive controls, we can now enable our development teams, and control our telemetry at runtime. Dialing up telemetry or increasing granularity when releasing a key new feature for example, or dialing up telemetry for a critical component during an incident. Real time visibility and feedback loops driven by MetaMetrics give you the real time cost impact of any changes.
And adaptive controls must meet you where you’re at, supporting the observability vendors and sources of data you already own, and do so in an open way as mentioned above. Furthermore, adaptive controls can aid with consolidations and migrations, even enabling you to accelerate the journey to OpenTelemetry instrumentation itself.
Summary
Controllability is the natural next step in the evolution of Observability—focused not just on seeing what’s happening, but taking action based on those insights. While Observability helps us understand the state of a system, Controllability enables us to shape that state in real time. At ControlTheory, this means:
- Cost Control: Regain visibility into where telemetry costs are coming from, and actively manage them using MetaMetrics, smart filtering, and open standards.
- Operational Control: Improve root cause analysis, compliance, and performance by filtering noise, elevating signal, and measuring what truly matters.
- Adaptive Control: Apply controls dynamically at runtime, empowering developers to instrument without fear and respond to changing conditions in real time.
Observability gave us visibility. Controllability gives us agency. It’s time to take back control.
press@controltheory.com